Learn to use adaptive algorithms to solve real-world streaming data problems. This book covers a multitude of data processing challenges, ranging from the simple to the complex. At each step, you will gain insight into real-world use cases, find solutions, explore code used to solve these problems, and create new algorithms for your own use.Authors Chanchal Chatterjee and Vwani P. Roychowdhury begin by introducing a common framework for creating adaptive algorithms, and demonstrating how to use it to address various streaming data issues. Examples range from using matrix functions to solve machine learning and data analysis problems to more critical edge computation problems. They handle time-varying, non-stationary data with minimal compute, memory, latency, and bandwidth.aUpon finishing this book, you will have a solid understanding of how to solve adaptive machine learning and data analytics problems and be able to derive new algorithms for your own use cases. You will also come away with solutions to high volume time-varying data with high dimensionality in a low compute, low latency environment.What You Will LearnO Apply adaptive algorithms to practical applications and examplesO Understand the relevant data representation features and computational models for time-varying multi-dimensional dataO Derive adaptive algorithms for mean,amedian, covariance, eigenvectors (PCA) and generalized eigenvectorsawith experiments on real dataO Speed up your algorithms and put them to use on real-world stationary and non-stationary dataO Master the applications of adaptive algorithms on critical edge device computation applicationsWho This Book Is ForMachine learning engineers, data scientist and architects, software engineers and architects handling edge device computation and data management.
Chapter 1. Introducing Data Representation FeaturesSet the context for the reader with important data representation features, present the need for adaptive algorithms to compute them and demonstrate how these algorithms are important in multiple disciplines. Additionally, discuss a common methodology adopted to derive all our algorithms.Sub-topics:1. Data representation features2. Computational models for time-varying multi-dimensional data3. Multi-disciplinary origin of adaptive algorithms4. Common Methodology for Derivations of Algorithms5. Outline of The Book Chapter 2. General Theories and NotationsIntroduce the reader to types of data in real-world streaming applications, discuss practical use cases and derive adaptive algorithms for mean, normalized mean, median, and covariances. Support the results with experiments on real data.Sub-topics:1. Introduction2. Stationary and Non-Stationary Sequences3. Use Cases for Algorithms Covered in this Chapter 4. Adaptive Mean and Covariance of Nonstationary Sequences5. Adaptive Covariance and Inverses6. Adaptive Normalized Mean Algorithm7. Adaptive Median Algorithm8. Experimental Results Chapter 3. Square Root and Inverse Square RootIntroduce readers to practical applications of square roots and inverse square roots of streaming data matrices, then present algorithms to compute them. Support the algorithms with real data.Sub-topics:1. Introduction and Use Cases2. Adaptive Square Root Algorithms3. Adaptive Inverse Square Root Algorithms4. Experimental Results Chapter 4. First Principal EigenvectorIntroduce the reader to adaptive computation of first principal component of streaming data, discuss the use cases with examples, derive ten algorithms with the common methodology adopted here. Demonstrate the algorithms with real-world non-stationary streaming data examples.Sub-topics:1. Introduction and Use Cases2. Algorithms and Objective Functions3. OJA Algorithm4. RQ, OJAN, and LUO Algorithms5. IT and XU Algorithms6. Penalty Function Algorithm 7. Augmented Lagrangian Algorithms8. Summary of Algorithms9. Experimental Results Chapter 5. Principal and Minor EigenvectorsIntroduce the reader to adaptive computation of all principal components, discuss powerful use cases with examples, derive 21 adaptive algorithms and demonstrate the algorithms on real-world time-varying data.Sub-topics:1. Introduction and Use Cases2. Algorithms and Objective Functions3. OJA Algorithms4. XU Algorithms5. PF Algorithms6. AL1 Algorithms7. AL2 Algorithms8. IT Algorithms9. RQ Algorithms10. Summary of Adaptive Eigenvector Algorithms11. Experimental Results Chapter 6. Accelerated Computation eigenvectorsIntroduce the reader to methods to speed up the adaptive algorithms presented in this book. Help the reader speed up a few algorithms and demonstrate their usefulness and acceleration on real-world stationery and non-stationary data.Sub-topics:1. Introduction2. Gradient Descent Algorithm3. Steepest Descent Algorithm4. Conjugate Direction Algorithm5. Newton-Raphson Algorithm6. Experimental Results Chapter 7. Generalized EigenvectorsIntroduce the reader to the adaptive computation of generalized eigenvectors of streaming data matrices in real-time applications. Discuss use cases and algorithms and show experimental results on real data.Sub-topics:1. Introduction and Use Cases2. Algorithms and Objective Functions3. OJA GEVD Algorithms4. XU GEVD Algorithms5. PF GEVD Algorithms6. AL1 GEVD Algorithms7. AL2 GEVD Algorithms 8. IT GEVD Algorithms9. RQ GEVD Algorithms10. Experimental Results Chapter 8. Real-World Applications Linear AlgorithmsHelp the reader understand real-world applications of the adaptive algorithms. Demonstrate five important applications of adaptive algorithms on critical edge device computation applications.Sub-topics:1. Detecting Feature Drift2. Adapt to Incoming Data Drift3. Compress High Volume Data4. Detecting Feature Anomalies
Accessing your eBook through Kortext
Once purchased, you can view your eBook through the Kortext app, available to download for Windows, Android and iOS devices. Once you have downloaded the app, your eBook will be available on your Kortext digital bookshelf and can even be downloaded to view offline anytime, anywhere, helping you learn without limits.
In addition, you'll have access to Kortext's smart study tools including highlighting, notetaking, copy and paste, and easy reference export.
To download the Kortext app, head to your device's app store or visit https://app.kortext.com to sign up and read through your browser.
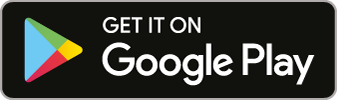
NB: eBook is only available for a single-user licence (i.e. not for multiple / networked users).